What Is the Value of Speaking the Same Language?
The NIH Policy for Data Management and Sharing requires researchers to plan for how scientific data will be preserved and shared. Researchers can leverage terminologies, ontologies, and other language approaches to comply with the required metadata and data standards elements of the plan. By applying common terminology to environmental health sciences data, researchers enhance its value by
- Enabling the assembly of datasets for computational modeling and knowledge discovery
- Facilitating consistent interpretation and understanding of data and metadata
- Increasing the findability of data
- Permitting integration and promoting interoperability of data and databases
- Supporting the transfer of knowledge between scientific communities
What Do We Mean by the “Same” Language?
First, let’s define language. Language is a system of communication consisting of symbols (sounds or written) and includes a set of rules for combining the symbols. Those rules include syntax (structure) and semantics (meaning). For example the sentence, “the chicken is ready to eat” is syntactically correct; however, it has a double meaning.
Semantics is the study of the interpretation and meaning of words and sentence structures. Linguistically, people rely on words having shared meanings to understand one another. Semantics is important because the meaning of words can change over time. For example, “cool” isn’t just used in the context of temperature but also now means being fashionable or hip. Terms that have high semantic complexity, e.g., “bank”, may have multiple, disagreeing definitions, which can result in miscommunication and misunderstanding. Does “bank” refer to a financial institution, land alongside a body of water, or a worktable used by carpenters? Even simple terms (e.g., mouse) can only be understood within a specific context.
As a result, it is beneficial for researchers to use a semantic standard aka a “common language” when describing their data and metadata. A common language is a community agreed-upon effort to define the acceptable meanings and uses of a word or phrase. A common language is most valuable when it has widespread adoption and consistent use among diverse groups. By using the same language, researchers can avoid ambiguity and misunderstandings, and facilitate integrating and analyzing their data.
What Are Examples of Common Language Approaches?
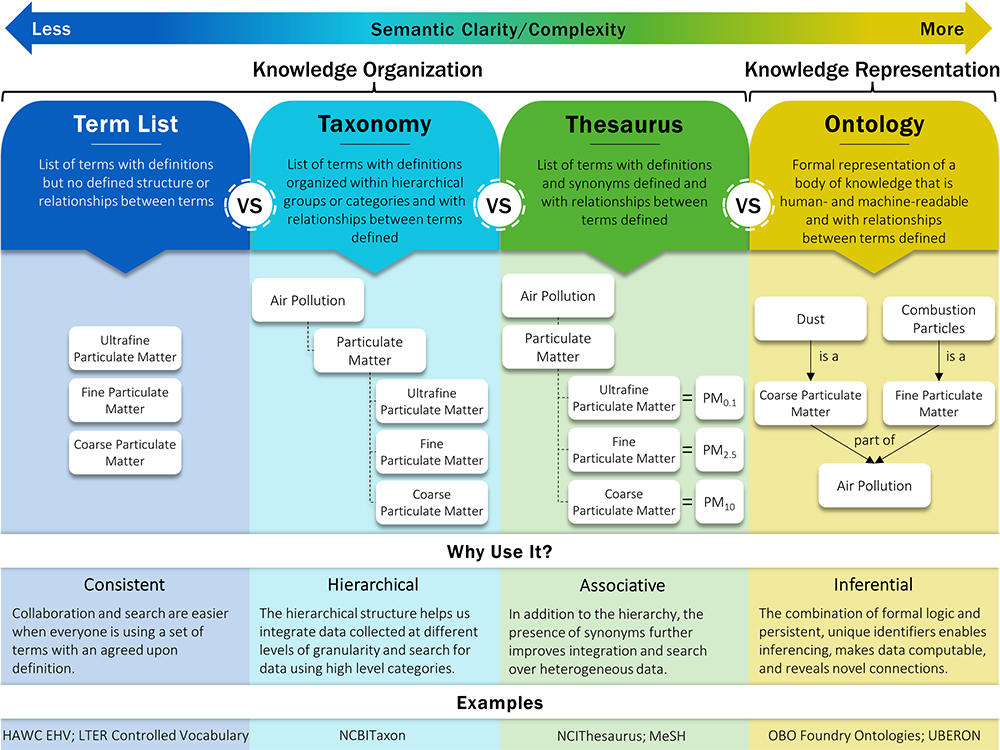
Different “flavors” of common language approaches exist and are often described as a type of knowledge organization system or used for knowledge representation. As you can see in the figure, approaches range from a simple term list and taxonomy to thesaurus and ontology. As one moves from left to right on the spectrum, semantic complexity increases, but so does the value gained by using that approach.
What Are the Uses of Common Language Types?
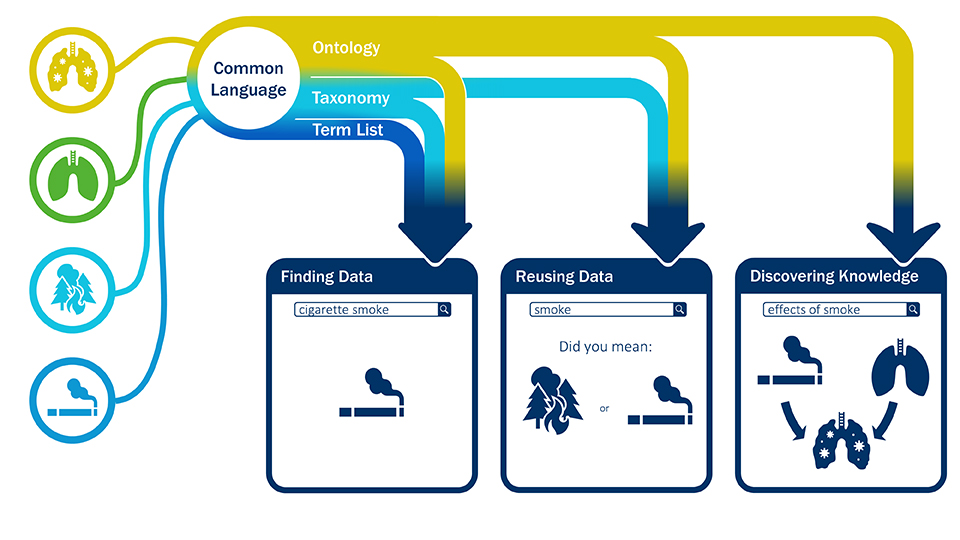
The following simplified research example (see figure) illustrates the value that each type of common language approach brings to data.
In our example, a researcher is studying the effects of cigarette smoke on lung cancer. At the very least, it would be beneficial for the researcher to use a list of self-defined standard terms to describe the data for her/his study. Think of a drop-down pick list that would ensure “cigarette smoke” is being consistently applied. The use of a term list enables the researcher to readily find and join data from her/his own experiments.
While a good starting point, this approach has its limits. If every researcher uses her/his own terms, then when data is made accessible for sharing, the ability to find and integrate the data would be impeded. Continuing the example, Researcher A describes the data using “cigarette smoke” while Researcher B uses “smoke.” If Researcher A wants to reuse Researcher B’s data, they don’t know if Researcher B means “cigarette” smoke or “wildfire” smoke. The lack of using a common term has created ambiguity and reduced understanding. A recommended best practice is to use a community-developed taxonomy or ontology to not only improve discoverability of the data but also ensure clear and consistent communication for data reuse.
The final scenario in our example considers how multiple smoke and lung cancer datasets can be integrated to discover new knowledge. Data system developers, modelers, among others utilize ontologies to create a structured representation of knowledge. This structured representation allows computer systems to understand the relationships and dependencies between concepts and entities enabling it to reason and make inferences or predictions on the data. Ontological terms that describe organ systems, disease, and exposures would enable inferences to be made on the research data. Given the value that ontologies provide, they are explored in more detail below.
What Are Ontologies?
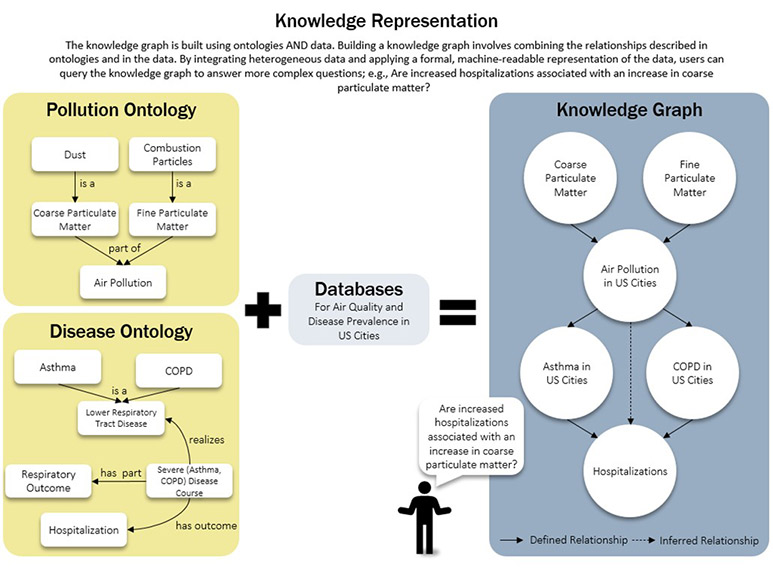
An ontology is a formal and explicit specification of a shared conceptualization of a domain of interest. It defines a set of concepts and categories along with their properties and relationships as well as any constraints on their usage. Ontologies are typically represented in a machine-readable format, such as OWL (Web Ontology Language) or RDF (Resource Description Framework).
What Is the Importance of an Ontology?
Because ontologies use a machine-readable format, they support automated reasoning, knowledge sharing, and semantic interoperability among different applications. As shown in the knowledge representation figure, by using concepts to integrate disparate information, users can now see connections and make inferences that they may not have otherwise. Ontologies are typically used to build knowledge graphs, which apply an ontological structure to describe real-world data. In the figure, the application of terms from the Pollution and Disease ontologies to air quality and disease prevalence data, enables a user to make inferences on the relationship between particulate matter and hospitalizations.
How Do I Incorporate Ontologies in My Work?
Even if you don’t want to create a knowledge graph, using ontologies rather than a thesaurus or taxonomy will be beneficial for others who may want to reuse your data. Users will be more readily able to integrate your data since you have used a community-supported standard to describe different domain areas.
Getting Started
- Check out the EHS Ontology Resources portal to find ontologies that may be relevant for your research and resources that can assist
- View recordings
- What Can Ontologies Do For You (21:52)
Jeanette Stingone presents perspective as an environmental epidemiologist working with other people’s data - Getting Started: How to Use Ontologies (33:18)
Dr. Ramona Walls presents on how to use ontologies for Superfund Research Center data when you have never used an ontology before.
- What Can Ontologies Do For You (21:52)