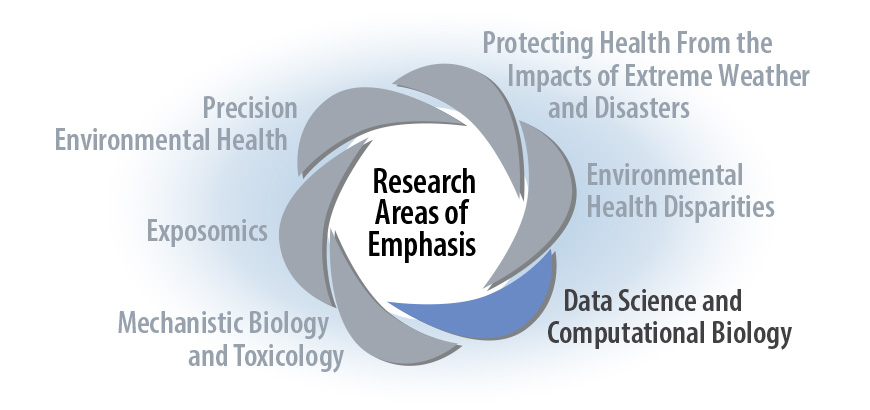
Data science and computational biology are increasingly foundational to conducting environmental health and biomedical research for the purpose of enhancing clinical care and public health outcomes. The development of innovative data science and data-driven approaches, such as integration of increasingly large and varied data types and data sources, holds enormous potential for advancing key research needs across the environmental health sciences. Promoting the expanded integration, harmonization, and use of available data through data science frameworks and Findable, Accessible, Interoperable, Reusable, and Computable (FAIR+) principles will facilitate such advances. AI is poised to expand the generation, use, and dissemination of environmental health knowledge in ways that will advance science while limiting misuse in order to increase public trust. NIEHS will continue to coordinate and partner with multidisciplinary groups and experts across the data science and computational biology communities. This will be done for the purpose of identifying activities to further NIH-wide priorities described in the NIH Strategic Plan for Data Science to capitalize on new discoveries and evolving data resources and approaches.
Translational Goal
Fully harness the increasing availability of varied environmental health-relevant data and advances in data science methods, tools, and management to enable more precise identification and understanding of the complex casual relationships between environmental exposures and health impacts and translation of scientific discovery into actionable know.
Priority Approaches
- Advance the integration and harmonization of large, complex data types from various sources and across distinct U.S. and global data ecosystems, including meteorological, geospatial, and health data.
- Advance the use and development of AI/ML and computational methods to construct and use cross-system (in vivo and in vitro), multi-scale, and interoperable models and knowledge platforms.
- Build AI/ML tools, training, and capacity to improve identification, use, and analysis of environmental health science, other biomedical, and sociobehavioral data.
- Develop, refine, and apply uniform data standards, common data elements, and standard curation methods to support FAIR+ principles and improve the validity and comparability of health studies and outcomes.