Introduction
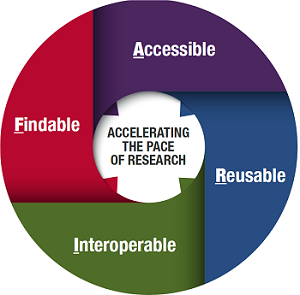
In 2019, SRP facilitated science-driven collaborative projects to enhance data integration, interoperability, and reuse. To accomplish these goals, SRP encouraged the applicants to develop “use cases” demonstrating where data management and data sharing could advance the interoperability and reuse of diverse and complex SRP data streams and increase the FAIR-ness of data.
Collaborators pursued rigorous research questions and identified current limitations to inform data management efforts for the SRP in the future. Together, the 19 projects utilized more than 50 datasets from SRP-funded research centers and individual research projects, external collaborators, and state, local, and federal sources.
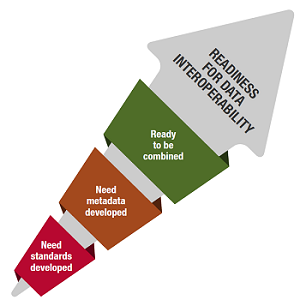
Teams starting at various stages along the spectrum of readiness for data interoperability worked together to set the groundwork to answer complex research environmental health questions that individual groups could not tackle alone.
Working closely with experts in data science, teams identified existing resources to advance FAIR-ness of SRP datasets and barriers to data sharing and interoperability.
For more details about each use case, including their innovative approaches to combining disparate datasets and creating user-friendly tools, the challenges teams experienced, and their recommendations to inform best practices for moving forward, please refer to the White Paper (2MB).
- Leveraging Data Across Human Populations: Researchers combined data from human populations to better understand the connections between exposure to hazardous substances and health.
- Integrating Omics Data Across Model Organisms: Leveraging large omics datasets, such as genomics and metabolomics, researchers sought to shed new light on the underlying molecular mechanisms by which hazardous substances affect health.
- Sharing Environmental Microbiome Data: By combining data on bacteria and microbes in the environment, researchers revealed how complex populations of microorganisms interact within an environment to provide useful information to improve strategies to remove hazardous substances from the environment.
- Geospatial Platforms and Visualization of Environmental Data: Researchers linked geospatial and other diverse datasets to create tools to visualize potential threats to human health.
- Data Sharing Tools, Workflows, and Platforms: Researchers sought to create tools, platforms, and strategies to make data more findable, accessible, interoperable, and reusable.